class: center, middle, inverse, title-slide # Introdução ao Machine Learning com R ###
### abril de 2021 --- # Ciência de dados <img src="static/img/ciclo-ciencia-de-dados.png" style = "display: block; margin-left: auto; margin-right: auto;"> --- # Referências .pull-left[ <a href = "http://www-bcf.usc.edu/~gareth/ISL/"> <img src="static/img/islr.png" style=" display: block; margin-left: auto; margin-right: auto;"></img> </a> ] .pull-right[ <a href = "https://web.stanford.edu/~hastie/Papers/ESLII.pdf"> <img src="static/img/esl.jpg" width = 58% style=" display: block; margin-left: auto; margin-right: auto;"></img> </a> ] --- # Referências .pull-left[ <a href = "https://r4ds.had.co.nz/"> <img src="static/img/r4ds.png" style=" display: block; margin-left: auto; margin-right: auto;"></img> </a> ] .pull-right[ <a href = "https://www.tidymodels.org/"> <img src="static/img/tidymodels.png" width = 75% style=" display: block; margin-left: auto; margin-right: auto;"></img> </a> ] --- class: middle, center, inverse # Introdução --- # O que é Machine Learning? <br> - Termo criado por Arthur Samuel, em 1959 <img src="static/img/arthur-sam.png" class="center2" width=100> - Machine Learning é o campo de estudo que dá aos computadores a habilidade de aprenderem sem serem explicitamente programados (Arthur Samuel, 1959). - Modelagem preditiva é um framework de análise de dados que visa gerar a estimativa mais precisa possível para uma quantidade ou fenômeno (Max Kuhn, 2014). <!-- --- --> <!-- ## O que é Machine Learning? --> <!-- **Objetivo**: Fazer com que computadores consigam contruir um programa (isso mesmo!) que replique o comportamento observado em exemplos, também chamados de **dados**. Esse programa normalmente é chamado de **modelo**. --> <!-- O procedimento que o computador executa para então estar apto a replicar o comportamento observado nos dados é usualmente chamado de **algoritmo**. --> <!-- Exemplo: O Fabeook usa um **algoritmo** para que um computador seja capaz de sugerir páginas interessantes para os seus usuários. Para tal, os **dados** são processados e o resultado final é um programa que, recebendo os dados de um usuário, devolve de quais páginas ele vai gostar. Nesse exemplo, o uso do **algoritmo** fez com que o computador construísse um **modelo**. --> --- # Motivação Somos consultores e fomos contratados para dar conselhos para uma empresa aumentar as suas vendas. Obtivemos o seguinte banco de dados <img src="01-intro-ml_files/figure-html/unnamed-chunk-3-1.png" style="display: block; margin: auto;" /> * PERGUNTA 1: Se eu investir `\(X_1\)` em propaganda numa certa mídia `\(X_2\)`, quanto vou vender, que vamos representar pela letra$Y$? --- # Motivação Somos consultores e fomos contratados para dar conselhos para uma empresa aumentar as suas vendas. Obtivemos o seguinte banco de dados <img src="01-intro-ml_files/figure-html/unnamed-chunk-4-1.png" style="display: block; margin: auto;" /> * PERGUNTA 1: Se eu investir `\(X_1\)` em propaganda numa certa mídia `\(X_2\)`, quanto vou vender, que vamos representar pela letra$Y$? * PERGUNTA 2: Como um computador pode captar essa relação? --- # Definições e Nomenclaturas ### A tabela por trás <table> <thead> <tr> <th style="text-align:left;"> midia </th> <th style="text-align:right;"> investimento </th> <th style="text-align:right;"> vendas </th> </tr> </thead> <tbody> <tr> <td style="text-align:left;"> TV </td> <td style="text-align:right;"> 220.3 </td> <td style="text-align:right;"> 24.7 </td> </tr> <tr> <td style="text-align:left;"> newspaper </td> <td style="text-align:right;"> 25.6 </td> <td style="text-align:right;"> 5.3 </td> </tr> <tr> <td style="text-align:left;"> newspaper </td> <td style="text-align:right;"> 38.7 </td> <td style="text-align:right;"> 18.3 </td> </tr> <tr> <td style="text-align:left;"> radio </td> <td style="text-align:right;"> 42.3 </td> <td style="text-align:right;"> 25.4 </td> </tr> <tr> <td style="text-align:left;"> radio </td> <td style="text-align:right;"> 43.9 </td> <td style="text-align:right;"> 22.3 </td> </tr> <tr> <td style="text-align:left;"> TV </td> <td style="text-align:right;"> 139.5 </td> <td style="text-align:right;"> 10.3 </td> </tr> <tr> <td style="text-align:left;"> radio </td> <td style="text-align:right;"> 11.0 </td> <td style="text-align:right;"> 7.2 </td> </tr> <tr> <td style="text-align:left;"> radio </td> <td style="text-align:right;"> 1.6 </td> <td style="text-align:right;"> 6.9 </td> </tr> </tbody> </table> --- # Machine Learning Se eu investir `\(X_1\)` em propaganda numa certa mídia `\(X_2\)`, quanto vou vender, que vamos representar pela letra$Y$? Para responder esse pergunta matematicamente, queremos encontrar uma função `\(f()\)`, e mais especificamente que o **computador** encontre, uma função tal que: <img src="static/img/y_fx.png" style="position: fixed; width: 40%; top: 325px; left: 300px;"> --- # Definições e Nomenclaturas * `\(X_1\)`, `\(X_2\)`, ..., `\(X_p\)`: variáveis explicativas (ou variáveis independentes ou *features* ou preditores). - `\(\boldsymbol{X} = {X_1, X_2, \dots, X_p}\)`: conjunto de todas as *features*. * __Y__: variável resposta (ou variável dependente ou *target*). * __Ŷ__: valor **esperado** (ou predição ou estimado ou *fitted*). * `\(f(X)\)` também é conhecida também como "Modelo" ou "Hipótese". ## No exemplo: - `\(X_1\)`: `midia` - indicadador de se a propaganda é para jornal, rádio, ou TV. - `\(X_2\)`: `investimento` - valor do orçamento * __Y__: `vendas` - qtd vendida --- # Exemplos de f(x) <img src="01-intro-ml_files/figure-html/unnamed-chunk-7-1.png" style="display: block; margin: auto;" /> --- # Exemplos de f(x) <img src="01-intro-ml_files/figure-html/unnamed-chunk-8-1.png" style="display: block; margin: auto;" /> --- # Por que ajustar uma f? * Predição * Inferência ## Inferência Em inferência estamos mais interessados em entender a relação entre as variáveis que conseguimos alterar (o investimento `\(X\)`) e a variável que queremos prever (a venda `\(Y\)`). Por exemplo: * Qual tipo de mídia influencia mais o `\(Y\)`? * Existe alguma mídia em que o investimento praticamente não faz efeito? Neste curso, vamos falar principalmente sobre **predição**. --- # Por que ajustar uma f? * Predição * Inferência ## Predição Em muitas situações `\(X\)` está disponível facilmente mas, `\(Y\)` não é fácil de descobrir. (Ou mesmo não é possível descobrí-lo). Então nós usamos `\(f(X)\)` como estimativa. Neste caso não importa se `\(f(X)\)` é uma reta ou uma escadinha desde que ela apresente predições boas para `\(Y\)`. --- background-image: url(static/img/usos_do_ml.png) background-position: left 140px background-size: contain # Por que ajustar uma f? --- # Definições e Nomenclaturas `\(f(X) \sim Y\)` quer dizer que `\(f(X)\)` está próximo de `\(Y\)`. Nós qualificamos essa aproximação comparando o dado observado com o dado esperado: - __Y__ é um valor **observado** (ou verdade ou *truth*) - __Ŷ__ é um valor **esperado** (ou predição ou estimado ou *fitted*). - __Y__ - __Ŷ__ é o resíduo (ou erro) Por definição, `\(\hat{Y} = f(x)\)` é o valor que a função `\(f\)` retorna. <img src="01-intro-ml_files/figure-html/unnamed-chunk-9-1.png" style="display: block; margin: auto;" /> --- # Definições e Nomenclaturas ### A tabela por trás <table> <thead> <tr> <th style="text-align:left;"> midia </th> <th style="text-align:right;"> investimento </th> <th style="text-align:right;"> vendas </th> <th style="text-align:right;"> arvore </th> <th style="text-align:right;"> regressao_linear </th> </tr> </thead> <tbody> <tr> <td style="text-align:left;"> TV </td> <td style="text-align:right;"> 220.3 </td> <td style="text-align:right;"> 24.7 </td> <td style="text-align:right;"> 18.1 </td> <td style="text-align:right;"> 17.8 </td> </tr> <tr> <td style="text-align:left;"> newspaper </td> <td style="text-align:right;"> 25.6 </td> <td style="text-align:right;"> 5.3 </td> <td style="text-align:right;"> 12.2 </td> <td style="text-align:right;"> 13.8 </td> </tr> <tr> <td style="text-align:left;"> newspaper </td> <td style="text-align:right;"> 38.7 </td> <td style="text-align:right;"> 18.3 </td> <td style="text-align:right;"> 14.9 </td> <td style="text-align:right;"> 14.4 </td> </tr> <tr> <td style="text-align:left;"> radio </td> <td style="text-align:right;"> 42.3 </td> <td style="text-align:right;"> 25.4 </td> <td style="text-align:right;"> 21.9 </td> <td style="text-align:right;"> 15.0 </td> </tr> <tr> <td style="text-align:left;"> radio </td> <td style="text-align:right;"> 43.9 </td> <td style="text-align:right;"> 22.3 </td> <td style="text-align:right;"> 16.8 </td> <td style="text-align:right;"> 15.1 </td> </tr> <tr> <td style="text-align:left;"> TV </td> <td style="text-align:right;"> 139.5 </td> <td style="text-align:right;"> 10.3 </td> <td style="text-align:right;"> 14.2 </td> <td style="text-align:right;"> 13.6 </td> </tr> <tr> <td style="text-align:left;"> radio </td> <td style="text-align:right;"> 11.0 </td> <td style="text-align:right;"> 7.2 </td> <td style="text-align:right;"> 12.2 </td> <td style="text-align:right;"> 13.4 </td> </tr> <tr> <td style="text-align:left;"> radio </td> <td style="text-align:right;"> 1.6 </td> <td style="text-align:right;"> 6.9 </td> <td style="text-align:right;"> 12.2 </td> <td style="text-align:right;"> 12.9 </td> </tr> </tbody> </table> --- # Métricas - quão boa é a aproximação de `\(f(x)\)`? Queremos medir o erro cometido por `\(f(x)\)`. Exemplo de medida de erro: **R**oot **M**ean **S**quared **E**rror. $$ RMSE = \sqrt{\frac{1}{N}\sum(y_i - \hat{y_i})^2} $$ Ou seja, nosso **objetivo** pode ser ## Encontrar `\(f(x)\)` que nos retorne o ~menor~ RMSE. --- # Métricas - quão boa é a aproximação de `\(f(x)\)`? Encontrar a reta que **erra menos** é um problema muito conhecido. Exemplo: Modelo de regressão linear `\(y = b + m x\)`, precisamos procurar os `\(b\)` e `\(m\)` que nos dão os melhores erros. 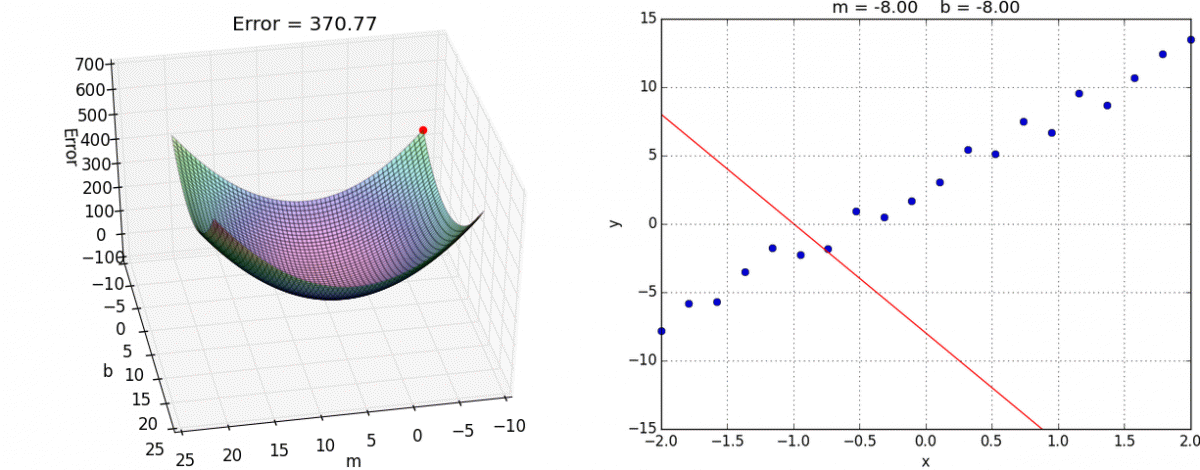 .footnote[ Fonte: [https://alykhantejani.github.io/images/gradient_descent_line_graph.gif](https://alykhantejani.github.io/images/gradient_descent_line_graph.gif) ] --- # Métricas - "Melhor f(x)" segundo o quê? Queremos a `\(f(x)\)` que **erre menos**. Exemplos de medida de erro: **R**oot **M**ean **S**quared **E**rror não é a única. $$ RMSE = \sqrt{\frac{1}{N}\sum(y_i - \hat{y_i})^2} $$ .pull-left[ MAE: Mean Absolute Error $$ MAE = \frac{1}{N}\sum|y_i - \hat{y_i}| $$ ] .pull-right[ R2: R-squared $$ R^2 = 1 - \frac{\sum(y_i - \color{salmon}{\hat{y_i}})^2}{\sum(y_i - \color{royalblue}{\bar{y}})^2} $$ ] --- ## Um pouco sobre R-quadrado ( `\(R^2\)` ) Muitas medidas de erro, que são gerais, são motivadas por ideias da regressão linear: $$ R^2 = 1 - \frac{\sum(y_i - \color{salmon}{\hat{y_i}})^2}{\sum(y_i - \color{royalblue}{\bar{y}})^2} = 1 - \frac{\color{salmon}{SQR}}{\color{royalblue}{SQT}} $$ .pull-left[ <img src="01-intro-ml_files/figure-html/unnamed-chunk-11-1.png" style="display: block; margin: auto;" /> ] .pull-right[ `\(R^2 \approx 1 \rightarrow \color{salmon}{SQR} << \color{royalblue}{SQT}\)`. `\(R^2 \approx 0 \rightarrow \color{salmon}{reta} \text{ em cima da } \color{royalblue}{reta}\)`. `\(R^2\)` pode ser calculado para qualquer tipo de modelo. ] .footnote[ Ver [ISL](https://www.ime.unicamp.br/~dias/Intoduction%20to%20Statistical%20Learning.pdf) página 68 (Assessing the Accuracy of the Model). ] --- # Flexibilidade ou Interpretabilidade de modelos de Machine Learning <img src="01-intro-ml_files/figure-html/unnamed-chunk-13-1.png" style="display: block; margin: auto;" /> --- # Overfitting (sobreajuste) Procurar a `\(f(x)\)` que se ajuste perfeitamente aos dados é uma boa estratégia? Intuição (TREINO: dados observados primeiro, TESTE: dados novos) 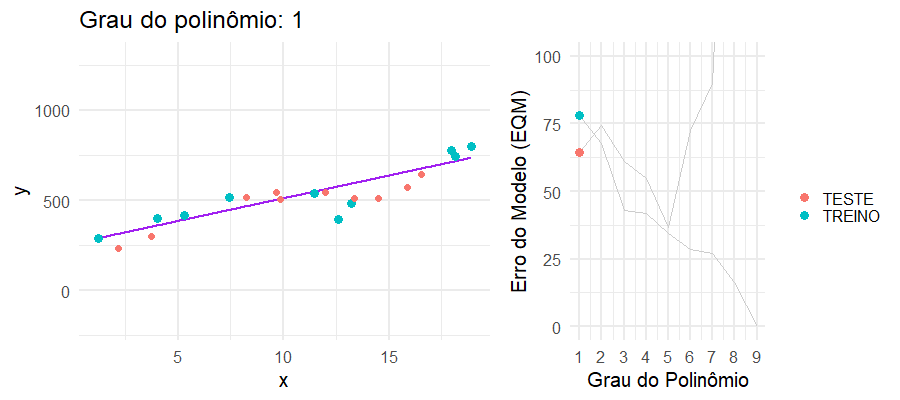 .footnote[ Ver [ISL](https://www.ime.unicamp.br/~dias/Intoduction%20to%20Statistical%20Learning.pdf) página 61 (Simple Linear Regression). ] --- # Overfitting (sobreajuste) <img src="01-intro-ml_files/figure-html/unnamed-chunk-15-1.png" width="720" style="display: block; margin: auto;" /> --- # Overfitting (sobreajuste) <img src="01-intro-ml_files/figure-html/unnamed-chunk-16-1.png" width="720" style="display: block; margin: auto;" /> --- # Overfitting (sobreajuste) <img src="01-intro-ml_files/figure-html/unnamed-chunk-17-1.png" width="720" style="display: block; margin: auto;" /> --- # Overfitting (sobreajuste) <img src="01-intro-ml_files/figure-html/unnamed-chunk-18-1.png" width="720" style="display: block; margin: auto;" /> --- # Dados novos vs antigos - **Base de Treino** (dados antigos): a base de histórico que usamos para ajustar o modelo. - **Base de Teste** (dados novos): a base que irá simular a chegada de dados novos, "em produção". .pull-left[ ```r initial_split(dados, prop=3/4) ``` > "Quanto mais complexo for o modelo, menor será o **erro de treino.**" > "Porém, o que importa é o **erro de teste**." ] .pull-right[ <img src="static/img/erro_treino_erro_teste.png" width = "500px"> ] --- # Dados novos vs antigos ## Estratégia ### 1) Separar inicialmente a base de dados em duas: treino e teste. ```r initial_split(dados, prop=3/4) # 3/4 da base será de treino ``` A base de teste que só será tocada quando a modelagem terminar. Ela nunca deverá influenciar as decisões que tomamos no período da modelagem. --- # Hiperparâmetros São parâmetros que têm que ser definidos antes de ajustar o modelo. Não há como achar o valor ótimo diretamente nas funções de custo. Precisam ser achados "na força bruta". Exemplo: `tree_depth` das árvores (profundidade das árvores) .pull-left[ ``` decision_tree(tree_depth = 2) ``` ] .pull-right[ <img src="01-intro-ml_files/figure-html/unnamed-chunk-21-1.png" style="display: block; margin: auto;" /> ] --- # Hiperparâmetros São parâmetros que têm que ser definidos antes de ajustar o modelo. Não há como achar o valor ótimo diretamente nas funções de custo. Precisam ser achados "na força bruta". Exemplo: `tree_depth` das árvores (profundidade das árvores) .pull-left[ ``` decision_tree(tree_depth = 5) ``` ] .pull-right[ <img src="01-intro-ml_files/figure-html/unnamed-chunk-22-1.png" style="display: block; margin: auto;" /> ] --- # Cross-validation (validação cruzada) **O que Validação cruzada faz:** estima (muito bem) o erro de predição. **Objetivo da Validação cruzada:** encontrar o melhor conjunto de hiperparâmetros. ## Estratégia .pull-left[ 1) Dividir o banco de dados em K partes. (Por ex, K = 5 como na figura) 2) Ajustar o mesmo modelo K vezes, deixar sempre um pedaço de fora para servir de base de teste. 3) Teremos K valores de erros de teste. Tira-se a média dos erros. ] .pull-right[ <img src="static/img/k-fold-cv.png"> ---------------------------------> linhas ] --- # Cross-validation (validação cruzada) ``` ## # 5-fold cross-validation ## # A tibble: 5 x 6 ## splits id n_treino n_teste regressao rmse_teste ## <list> <chr> <dbl> <dbl> <list> <dbl> ## 1 <split [40/10]> Fold1 40 10 <lm> 12.0 ## 2 <split [40/10]> Fold2 40 10 <lm> 21.4 ## 3 <split [40/10]> Fold3 40 10 <lm> 16.6 ## 4 <split [40/10]> Fold4 40 10 <lm> 11.3 ## 5 <split [40/10]> Fold5 40 10 <lm> 13.8 ``` ERRO DE VALIDAÇÃO CRUZADA: `$$RMSE_{cv} = \sqrt{\frac{1}{5}\sum_{i=1}^{5}RMSE_{Fold_i}} = 3,88$$` --- # Cross-validation (validação cruzada) ### Esquema das divisões de bases: <img src="static/img/resampling.svg" width = 75%> .footnote[ Fonte: [bookdown.org/max/FES/resampling.html](https://bookdown.org/max/FES/resampling.html) ] --- class: inverse background-image: url(static/img/ml_101.png) background-position: left 140px background-size: contain # Resumo dos conceitos --- <img src="https://wordstream-files-prod.s3.amazonaws.com/s3fs-public/styles/simple_image/public/images/machine-learning1.png?Q_SmWhhhAEOO_32HNjPhcxsPhreWV26o&itok=yjEJbEKD" style="display: block; margin-left: auto; margin-right: auto;"></img> --- # Regressão Linear .pull-left[ ### Regressão Linear Simples $$ y = \beta_0 + \beta_1x $$ ### Exemplo: $$ dist = \beta_0 + \beta_1speed $$ ] .pull-right[ <img src="01-intro-ml_files/figure-html/unnamed-chunk-25-1.png" style="display: block; margin: auto;" /> ] ### No R: ```r linear_reg() %>% fit(dist ~ speed, data=cars) ``` .footnote[ Ver [ISL](https://www.ime.unicamp.br/~dias/Intoduction%20to%20Statistical%20Learning.pdf) página 61 (Simple Linear Regression). ] --- # Regressão Linear .pull-left[ ### Regressão Linear Múltipla $$ y = \beta_0 + \beta_1x_1 + \dots + \beta_px_p $$ ### Exemplo: $$ mpg = \beta_0 + \beta_1wt + \beta_2disp $$ ] .pull-right[
] ### No R: ```r linear_reg() %>% fit(disp ~ wt + mpg, data=mtcars) ``` .footnote[ Fonte: [sthda.com/impressive-package-for-3d](http://www.sthda.com/english/wiki/impressive-package-for-3d-and-4d-graph-r-software-and-data-visualization) ] --- # Regressão Linear - "Melhor Reta" Queremos a reta que **erre menos**. Modelo: `\(y = b + m x\)` 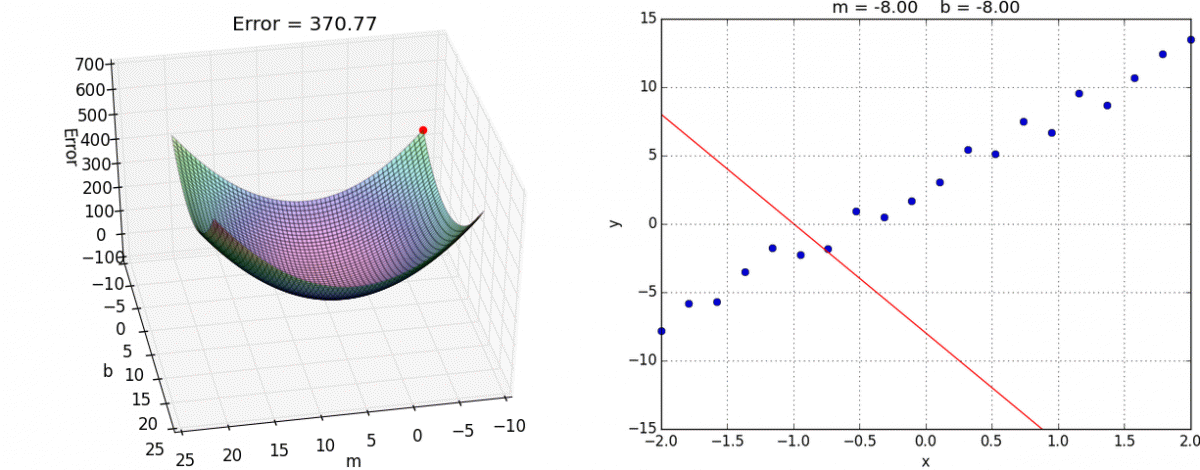 .footnote[ Fonte: [https://alykhantejani.github.io/images/gradient_descent_line_graph.gif](https://alykhantejani.github.io/images/gradient_descent_line_graph.gif) ] --- # Regressão Linear - "Melhor Reta" Queremos a reta que **erre menos**. Uma medida de erro: RMSE $$ RMSE = \sqrt{\frac{1}{N}\sum(y_i - \hat{y_i})^2} $$ Ou seja, nosso é **encontrar os `\(\hat{\beta}'s\)` que nos retorne o ~menor~ RMSE.** #### IMPORTANTE! o RMSE é a nossa **Função de Custo** e pode ser diferente da **Métrica** que vimos nos slides anteriores! - **Função de Custo** - para encontrar os melhores parâmetros. - **Métrica** - para encontrar os melhores hiperparâmetros. --- ## Qual o valor ótimo para `\(\beta_0\)` e `\(\beta_1\)`? No nosso exemplo, a nossa **HIPÓTESE** é de que $$ dist = \beta_0 + \beta_1speed $$ Então podemos escrever o RMSE $$ RMSE = \sqrt{\frac{1}{N}\sum(y_i - \hat{y_i})^2} = \sqrt{\frac{1}{N}\sum(y_i - \color{red}{(\hat{\beta}_0 + \hat{\beta}_1speed)})^2} $$ .pull-left[ Método mais utilizado para otimizar modelos com parâmetros: **Gradient Descent** Ver [Wikipedia do Gradient Descent](https://en.wikipedia.org/wiki/Gradient_descent) ] .pull-right[ <img src = "static/img/gradient_descent.png" width = 65%> ] --- ## Qual o valor ótimo para `\(\beta_0\)` e `\(\beta_1\)`? No nosso exemplo, a nossa **HIPÓTESE** é de que $$ dist = \beta_0 + \beta_1speed $$ Então podemos escrever o RMSE $$ RMSE = \sqrt{\frac{1}{N}\sum(y_i - \hat{y_i})^2} = \sqrt{\frac{1}{N}\sum(y_i - \color{red}{(\hat{\beta}_0 + \hat{\beta}_1speed)})^2} $$ Curiosidade: com ajuda do Cálculo é possível mostrar que os valores ótimos para `\(\beta_0\)` e `\(\beta_1\)` são `\(\hat{\beta}_1 = \frac{\sum(x_i - \bar{x})(y_i - \bar{y})}{\sum(x_i - \bar{x})^2}\)` `\(\hat{\beta}_0 = \bar{y} - \hat{\beta}_1\bar{x}\)` OBS: Já que vieram do EQM, eles são chamados de **Estimadores de Mínimos Quadrados**. --- ## Depois de estimar... $$ \hat{y} = \hat{\beta}_0 + \hat{\beta}_1x $$ ### Exemplo: $$ \hat{dist} = \hat{\beta}_0 + \hat{\beta}_1speed $$ Colocamos um `\(\hat{}\)` em cima dos termos para representar "estimativas". Ou seja, `\(\hat{y}_i\)` é uma estimativa de `\(y_i\)`. No nosso exemplo, - `\(\hat{\beta}_0\)` é uma estimativa de `\(\beta_0\)` e vale `-17.579`. - `\(\hat{\beta}_1\)` é uma estimativa de `\(\beta_1\)` e vale `3.932`. - `\(\hat{dist}\)` é uma estimativa de `\(dist\)` e vale `-17.579 + 3.932 x speed`. ```r # Exercício: se speed for 15 m/h, quanto que # seria a distância dist esperada? ``` --- ## Interpretação dos parâmetros .pull-left[ <img src="01-intro-ml_files/figure-html/unnamed-chunk-30-1.png" style="display: block; margin: auto;" /> $$ y = \color{darkgblue}{\beta_0} + \color{darkgreen}{\beta_1}x $$ ] .pull-right[ ### Interpretações matemáticas `\(\color{darkgblue}{\beta_0}\)` é o lugar em que a reta cruza o eixo Y. `\(\color{darkgreen}{\beta_1}\)` é a derivada de Y em relação ao X. É quanto Y varia quando X varia em 1 unidade. ### Interpretações estatísticas `\(\color{darkgblue}{\beta_0}\)` é a distância percorrida esperada quando o carro está parado (X = 0). `\(\color{darkgreen}{\beta_1}\)` é o efeito médio na distância por variar 1 ml/h na velocidade do carro. ] --- ## Regularização - LASSO Relembrando o nossa **função de custo** RMSE. `$$RMSE = \sqrt{\frac{1}{N}\sum(y_i - \hat{y_i})^2} = \sqrt{\frac{1}{N}\sum(y_i - \color{red}{(\hat{\beta}_0 + \hat{\beta}_1x_{1i} + \dots + \hat{\beta}_px_{pi})})^2}$$` Regularizar é "não deixar os `\(\beta's\)` soltos demais". `$$RMSE_{regularizado} = RMSE + \color{red}{\lambda}\sum_{j = 1}^{p}|\beta_j|$$` Ou seja, **penalizamos** a função de custo se os `\(\beta's\)` forem muito grandes. **PS1:** O `\(\color{red}{\lambda}\)` é um hiperparâmetro para a Regressão Linear. **PS2:** Quanto maior o `\(\color{red}{\lambda}\)`, mais penalizamos os `\(\beta's\)` por serem grandes. .footnote[ Ver [ISL](https://www.ime.unicamp.br/~dias/Intoduction%20to%20Statistical%20Learning.pdf) página 203 (Linear Model Selection and Regularization). ] --- ## Regularização - LASSO Existe um `\(\color{red}{\lambda}\)` que retorna o menor erro de cross-validation. 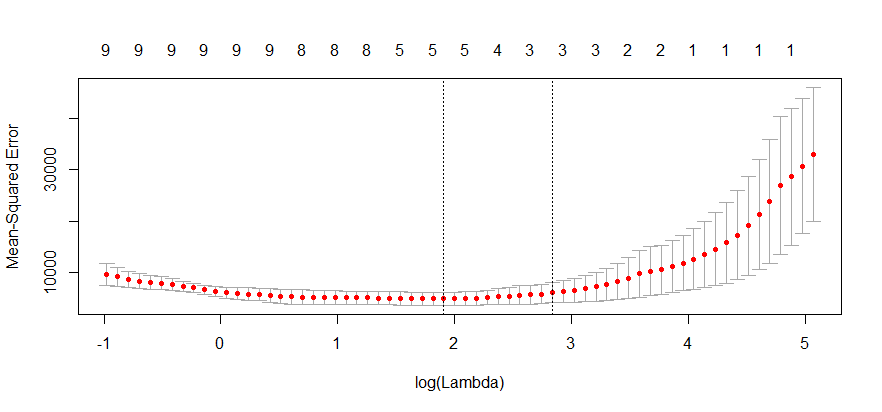 .footnote[ Ver [ISL](https://www.ime.unicamp.br/~dias/Intoduction%20to%20Statistical%20Learning.pdf) página 219 (The LASSO). ] --- ## Regularização - LASSO Conforme aumentamos o `\(\color{red}{\lambda}\)`, forçamos os `\(\beta's\)` a serem cada vez menores. 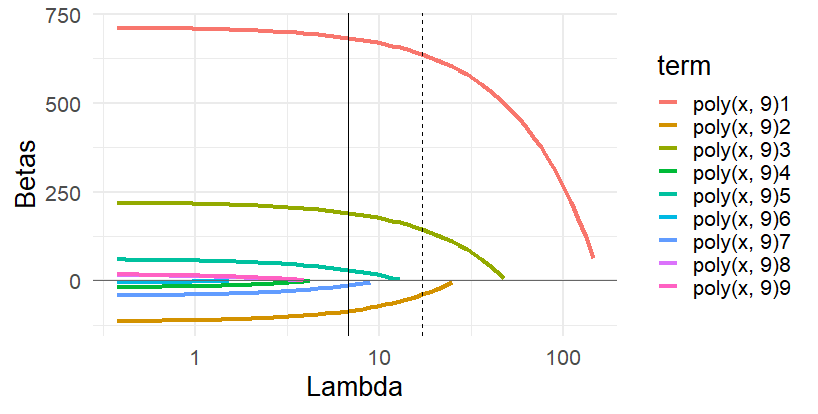 .footnote[ Ver [ISL](https://www.ime.unicamp.br/~dias/Intoduction%20to%20Statistical%20Learning.pdf) página 219 (The LASSO). ] --- ## Ir para o R --- ## Preditores Categóricos ### Preditor com apenas 2 categorias Saldo médio no cartão de crédito é diferente entre homens e mulheres? <img src="01-intro-ml_files/figure-html/unnamed-chunk-31-1.png" style="display: block; margin: auto;" /> ```r summary(lm(Balance ~ Gender, data = Credit)) # Coefficients: # Estimate Std.Error t value Pr(>|t|) # (Intercept) 509.80 33.13 15.389 <2e-16 *** # GenderFemale 19.73 46.05 0.429 0.669 ``` .footnote[ Ver [ISL](https://www.ime.unicamp.br/~dias/Intoduction%20to%20Statistical%20Learning.pdf) página 84 (Predictors with Only Two Levels). ] --- ## Preditores Categóricos ### Preditor com apenas 2 categorias Saldo médio no cartão de crédito é diferente entre homens e mulheres? <img src="01-intro-ml_files/figure-html/unnamed-chunk-33-1.png" style="display: block; margin: auto;" /> $$ y_i = \beta_0 + \beta_1x_i \space\space\space\space\space\space \text{em que}\space\space\space\space\space\space x_i = \Bigg\\{\begin{array}{ll}1&\text{se a i-ésima pessoa for }\texttt{female}\\\\ 0&\text{se a i-ésima pessoa for } \texttt{male}\end{array} $$ .footnote[ Ver [ISL](https://www.ime.unicamp.br/~dias/Intoduction%20to%20Statistical%20Learning.pdf) página 84 (Predictors with Only Two Levels). ] --- ## Preditores Categóricos ### Preditor com 3 ou mais categorias .pull-left[ <img src="01-intro-ml_files/figure-html/unnamed-chunk-34-1.png" style="display: block; margin: auto;" /> ```r summary(lm(Balance ~ Ethnicity, data = Credit)) # Estimate Std.Error t value Pr(>|t|) # (Intercept) 531.00 46.32 11.464 <2e-16 *** # EthnicityAsian -18.69 65.02 -0.287 0.774 # EthnicityCaucasian -12.50 56.68 -0.221 0.826 ``` ] .pull-right[ Modelo `$$y_i = \beta_0 + \beta_1x_{1i} + \beta_2x_{2i}$$` Em que `\(x_{1i} = \Bigg \{ \begin{array}{ll} 1 & \text{se for }\texttt{Asian}\\0&\text{caso contrário}\end{array}\)` `\(x_{2i} = \Bigg \{ \begin{array}{ll} 1 & \text{se for }\texttt{Caucasian}\\0&\text{caso contrário}\end{array}\)` ] --- ## Preditores Categóricos ### Preditor com 3 ou mais categorias "One hot enconding" ou "Dummies" ou "Indicadores". <table> <thead> <tr> <th style="text-align:left;"> Ethnicity </th> <th style="text-align:right;"> (Intercept) </th> <th style="text-align:right;"> EthnicityAsian </th> <th style="text-align:right;"> EthnicityCaucasian </th> </tr> </thead> <tbody> <tr> <td style="text-align:left;"> Caucasian </td> <td style="text-align:right;"> 1 </td> <td style="text-align:right;"> 0 </td> <td style="text-align:right;"> 1 </td> </tr> <tr> <td style="text-align:left;"> Asian </td> <td style="text-align:right;"> 1 </td> <td style="text-align:right;"> 1 </td> <td style="text-align:right;"> 0 </td> </tr> <tr> <td style="text-align:left;"> Asian </td> <td style="text-align:right;"> 1 </td> <td style="text-align:right;"> 1 </td> <td style="text-align:right;"> 0 </td> </tr> <tr> <td style="text-align:left;"> Asian </td> <td style="text-align:right;"> 1 </td> <td style="text-align:right;"> 1 </td> <td style="text-align:right;"> 0 </td> </tr> <tr> <td style="text-align:left;"> Caucasian </td> <td style="text-align:right;"> 1 </td> <td style="text-align:right;"> 0 </td> <td style="text-align:right;"> 1 </td> </tr> <tr> <td style="text-align:left;"> Caucasian </td> <td style="text-align:right;"> 1 </td> <td style="text-align:right;"> 0 </td> <td style="text-align:right;"> 1 </td> </tr> <tr> <td style="text-align:left;"> African American </td> <td style="text-align:right;"> 1 </td> <td style="text-align:right;"> 0 </td> <td style="text-align:right;"> 0 </td> </tr> <tr> <td style="text-align:left;"> Asian </td> <td style="text-align:right;"> 1 </td> <td style="text-align:right;"> 1 </td> <td style="text-align:right;"> 0 </td> </tr> </tbody> </table> steps: `step_dummy()` --- ## Preditores Categóricos ### Preditor com 3 ou mais categorias Interpretação dos parâmetros: `\(y_{i} = \left\{ \begin{array}{ll} \beta_0 & \text{se for }\texttt{Afro American}\\ \beta_0 + \beta_1&\text{se for } \texttt{Asian}\\ \beta_0 + \beta_2&\text{se for } \texttt{Caucasian}\end{array}\right.\)` ```r # interprete cada um dos três parâmetros individualmente. ``` --- ## Principais problemas dos modelos lineares ### Vamos estudar nesse curso - Escala das variáveis explicativas (apenas LASSO sofre) - Relação não-linear entre X e Y - Outliers/Pontos de alavanca ### Não vamos estudar nesse curso - Heterocedasticidade: Variância dos erros não constantes - Multicolinearidade (LASSO resolve) - Correlação entre os erros (séries temporais, por ex.) --- ## Transformações Não Lineares dos Preditores ### Exemplo: log .pull-left[ Modelo real: `\(y = 10 + 0.5log(x)\)` ] .pull-right[ Modelo proposto: `\(\small y = \beta_0 + \beta_1log(x)\)` ] Outras transformações comuns: raíz quadrada, Box-Cox. steps: `step_log()`, `step_BoxCox()`, `step_sqrt()` --- ## Transformações Não Lineares dos Preditores ### Exemplo: Regressão Polinomial .pull-left[ Modelo real: `\(y = 500 + 0.4(x-10)^3\)` ] .pull-right[ Modelo proposto: `\(y = \beta_0 + \beta_1x + \beta_2x^2 + \beta_3x^3\)` ] <img src="01-intro-ml_files/figure-html/unnamed-chunk-40-1.png" style="display: block; margin: auto;" /> Outras expansões comuns: b-splines, natural splines. steps: `step_poly()`, `step_bs()`, `step_ns` --- ## Interações Modelo proposto (Matemático): Seja `Sepal.Width` o `\(y\)` e `Sepal.Length` o `\(x\)`, `$$\small \begin{array}{l} y = \beta_0 + \beta_1x + \beta_2I_{versicolor} + \beta_3I_{virginica} + \beta_4\color{red}{xI_{versicolor}} + \beta_5\color{red}{xI_{virginica}}\end{array}$$` <img src="01-intro-ml_files/figure-html/unnamed-chunk-41-1.png" height="260" style="display: block; margin: auto;" /> step: `step_interact(terms = ~ Sepal.Length:Species)` --- ## Outliers <img src="01-intro-ml_files/figure-html/unnamed-chunk-42-1.png" style="display: block; margin: auto;" /> .footnote[ Ver [ISL](https://www.ime.unicamp.br/~dias/Intoduction%20to%20Statistical%20Learning.pdf) página 96 (Outliers). ] --- ## Outliers <img src="01-intro-ml_files/figure-html/unnamed-chunk-43-1.png" style="display: block; margin: auto;" /> .footnote[ Ver [ISL](https://www.ime.unicamp.br/~dias/Intoduction%20to%20Statistical%20Learning.pdf) página 96 (Outliers). ] --- ## Outliers <img src="01-intro-ml_files/figure-html/unnamed-chunk-44-1.png" style="display: block; margin: auto;" /> .footnote[ Ver [ISL](https://www.ime.unicamp.br/~dias/Intoduction%20to%20Statistical%20Learning.pdf) página 96 (Outliers). ] --- ## Outliers ### Diagnóstico - Visualização univariada (histograma, boxplot); - Comparação do valor com o desvio padrão; ### Tratamentos - Transformações log(), etc; - Categorização; - Remover os valores extremos (raramente boa ideia); - Usar Random Forest/XGBoost; - Usar MAE em vez de RMSE; --- ## Ir para o R --- class: inverse, center, middle # CLASSIFICAÇÃO --- # Regressão Logística .pull-left[ ### Para `\(Y \in \{0, 1\}\)` (binário) $$ log\left\(\frac{p}{1-p}\right\) = \beta_0 + \beta_1x $$ em que `\(p = P(Y = 1|x)\)`. Ou... $$ p = \frac{1}{1 + e^{-(\beta_0 + \beta_1x)}} $$ ] .pull-right[ <img src="01-intro-ml_files/figure-html/unnamed-chunk-45-1.png" style="display: block; margin: auto;" /> ] ### No R: ```r logistic_reg() %>% fit(dist ~ speed, data=Default) ``` .footnote[ Ver [ISL](https://www.ime.unicamp.br/~dias/Intoduction%20to%20Statistical%20Learning.pdf) página 131 (Logistic Regression). ] --- # Regressão Logística <img src="01-intro-ml_files/figure-html/unnamed-chunk-47-1.png" style="display: block; margin: auto;" /> --- # Regressão Logística
--- # Árvore de Decisão
--- # Regressão Logística - Custo e Regularização A **função de custo** da Regressão Logística chama-se *log-loss* (ou *deviance* ou *Binary Cross-Entropy*): `$$D = \frac{-1}{N}\sum[y_i \log\hat{y_i} + (1 - y_i )\log(1 - \hat{y_i})]$$` --- # Regressão Logística - Custo e Regularização A **função de custo** da Regressão Logística chama-se *log-loss* (ou *deviance* ou *Binary Cross-Entropy*): `$$D = \frac{-1}{N}\sum[y_i \log\hat{y_i} + (1 - y_i )\log(1 - \hat{y_i})]$$` Para cada linha da base de dados seria assim... `$$D_i = \begin{cases} -\log(\hat{y}_i) & \text{quando} \space y_i = 1 \\\\ -\log(1-\hat{y}_i) & \text{quando} \space y_i = 0 \end{cases}$$` <img src="01-intro-ml_files/figure-html/unnamed-chunk-50-1.png" style="display: block; margin: auto;" /> --- # Regressão Logística - Custo e Regularização A **função de custo** da Regressão Logística chama-se *log-loss* (ou *deviance* ou *Binary Cross-Entropy*): `$$D = \frac{-1}{N}\sum[y_i \log\hat{y_i} + (1 - y_i )\log(1 - \hat{y_i})]$$` Regularizar é analogo a Regressão Linear. `$$D_{regularizado} = D + \color{red}{\lambda}\sum_{j = 1}^{p}|\beta_j|$$` **PS1:** `\(\hat{y_i} = \hat{p_i} = \hat{P}(Y = 1|X)\)`. **PS2:** Se `\(\log\left(\frac{\hat{p_i}}{1-\hat{p_i}}\right) = \beta_0 + \beta_1x\)` então `\(\hat{p_i} = \frac{1}{1 + e^{-(\beta_0 + \beta_1x)}}\)`. --- # Regressão Logística - Predições O "produto final" será um vetor de probabilidades estimadas. .pull-left[ <table> <thead> <tr> <th style="text-align:right;background-color: white !important;text-align: center;"> pts excl </th> <th style="text-align:left;background-color: white !important;text-align: center;"> classe observada </th> <th style="text-align:right;background-color: white !important;text-align: center;"> prob </th> <th style="text-align:left;background-color: white !important;text-align: center;"> classe predita </th> </tr> </thead> <tbody> <tr> <td style="text-align:right;background-color: white !important;text-align: center;"> 167 </td> <td style="text-align:left;background-color: white !important;text-align: center;"> Spam </td> <td style="text-align:right;background-color: white !important;text-align: center;"> 0.79 </td> <td style="text-align:left;background-color: white !important;text-align: center;"> Spam </td> </tr> <tr> <td style="text-align:right;background-color: white !important;text-align: center;"> 129 </td> <td style="text-align:left;background-color: white !important;text-align: center;"> Spam </td> <td style="text-align:right;background-color: white !important;text-align: center;"> 0.45 </td> <td style="text-align:left;background-color: white !important;text-align: center;"> Não Spam </td> </tr> <tr> <td style="text-align:right;background-color: white !important;text-align: center;"> 299 </td> <td style="text-align:left;background-color: white !important;text-align: center;"> Spam </td> <td style="text-align:right;background-color: white !important;text-align: center;"> 1.00 </td> <td style="text-align:left;background-color: white !important;text-align: center;"> Spam </td> </tr> <tr> <td style="text-align:right;background-color: white !important;text-align: center;"> 270 </td> <td style="text-align:left;background-color: white !important;text-align: center;"> Spam </td> <td style="text-align:right;background-color: white !important;text-align: center;"> 1.00 </td> <td style="text-align:left;background-color: white !important;text-align: center;"> Spam </td> </tr> <tr> <td style="text-align:right;background-color: white !important;text-align: center;"> 187 </td> <td style="text-align:left;background-color: white !important;text-align: center;"> Spam </td> <td style="text-align:right;background-color: white !important;text-align: center;"> 0.89 </td> <td style="text-align:left;background-color: white !important;text-align: center;"> Spam </td> </tr> <tr> <td style="text-align:right;background-color: white !important;text-align: center;"> 85 </td> <td style="text-align:left;background-color: white !important;text-align: center;"> Não Spam </td> <td style="text-align:right;background-color: white !important;text-align: center;"> 0.12 </td> <td style="text-align:left;background-color: white !important;text-align: center;"> Não Spam </td> </tr> </tbody> </table> ] .pull-right[ <img src="01-intro-ml_files/figure-html/unnamed-chunk-52-1.png" style="display: block; margin: auto;" /> ] --- # Matriz de Confusão .pull-left[ <table class="table table-bordered" style="font-size: 20px; width: auto !important; margin-left: auto; margin-right: auto;"> <thead> <tr> <th style="empty-cells: hide;border-bottom:hidden;" colspan="1"></th> <th style="border-bottom:hidden;padding-bottom:0; padding-left:3px;padding-right:3px;text-align: center; padding-right: 4px; padding-left: 4px; background-color: white !important;" colspan="2"><div style="border-bottom: 1px solid #ddd; padding-bottom: 5px; ">Observado</div></th> </tr> <tr> <th style="text-align:left;background-color: white !important;text-align: center;"> Predito </th> <th style="text-align:left;background-color: white !important;text-align: center;"> Neg </th> <th style="text-align:left;background-color: white !important;text-align: center;"> Pos </th> </tr> </thead> <tbody> <tr> <td style="text-align:left;background-color: white !important;text-align: center;width: 3in; font-weight: bold;"> Neg </td> <td style="text-align:left;background-color: white !important;text-align: center;width: 3in; "> TN </td> <td style="text-align:left;background-color: white !important;text-align: center;width: 2in; "> FN </td> </tr> <tr> <td style="text-align:left;background-color: white !important;text-align: center;width: 3in; font-weight: bold;"> Pos </td> <td style="text-align:left;background-color: white !important;text-align: center;width: 3in; "> FP </td> <td style="text-align:left;background-color: white !important;text-align: center;width: 2in; "> TP </td> </tr> </tbody> </table> <br/> <table class="table table-bordered" style="font-size: 20px; width: auto !important; margin-left: auto; margin-right: auto;"> <thead> <tr> <th style="border-bottom:hidden;padding-bottom:0; padding-left:3px;padding-right:3px;text-align: center; color: red !important;padding-right: 4px; padding-left: 4px; background-color: white !important;" colspan="1"><div style="border-bottom: 1px solid #ddd; padding-bottom: 5px; ">p > 50%</div></th> <th style="border-bottom:hidden;padding-bottom:0; padding-left:3px;padding-right:3px;text-align: center; color: black !important;padding-right: 4px; padding-left: 4px; background-color: white !important;" colspan="2"><div style="border-bottom: 1px solid #ddd; padding-bottom: 5px; ">Observado</div></th> </tr> <tr> <th style="text-align:left;background-color: white !important;text-align: center;"> Predito </th> <th style="text-align:right;background-color: white !important;text-align: center;"> Não Spam </th> <th style="text-align:right;background-color: white !important;text-align: center;"> Spam </th> </tr> </thead> <tbody> <tr> <td style="text-align:left;background-color: white !important;text-align: center;width: 3in; font-weight: bold;"> Não Spam </td> <td style="text-align:right;background-color: white !important;text-align: center;width: 3in; "> 410 </td> <td style="text-align:right;background-color: white !important;text-align: center;width: 2in; "> 73 </td> </tr> <tr> <td style="text-align:left;background-color: white !important;text-align: center;width: 3in; font-weight: bold;"> Spam </td> <td style="text-align:right;background-color: white !important;text-align: center;width: 3in; "> 52 </td> <td style="text-align:right;background-color: white !important;text-align: center;width: 2in; "> 465 </td> </tr> </tbody> </table> ] .pull-right[ $$ \begin{array}{lcc} \mbox{accuracy} & = & \frac{TP + TN}{TP + TN + FP + FN}\\\\ & & \\\\ \mbox{precision} & = & \frac{TP}{TP + FP}\\\\ & & \\\\ \mbox{recall/TPR} & = & \frac{TP}{TP + FN} \\\\ & & \\\\ \mbox{F1 score} & =& \frac{2}{1/\mbox{precision} + 1/\mbox{recall}}\\\\ & & \\\\ \mbox{FPR} & = & \frac{FP}{FP + TN} \end{array}` $$ ] --- # Nota de Corte (Threshold) .pull-left[ <table class="table table-bordered" style="font-size: 16px; width: auto !important; margin-left: auto; margin-right: auto;"> <thead> <tr> <th style="border-bottom:hidden;padding-bottom:0; padding-left:3px;padding-right:3px;text-align: center; color: red !important;padding-right: 4px; padding-left: 4px; background-color: white !important;" colspan="1"><div style="border-bottom: 1px solid #ddd; padding-bottom: 5px; ">p > 10%</div></th> <th style="border-bottom:hidden;padding-bottom:0; padding-left:3px;padding-right:3px;text-align: center; color: black !important;padding-right: 4px; padding-left: 4px; background-color: white !important;" colspan="2"><div style="border-bottom: 1px solid #ddd; padding-bottom: 5px; ">Observado</div></th> </tr> <tr> <th style="text-align:left;background-color: white !important;text-align: center;"> Predito </th> <th style="text-align:right;background-color: white !important;text-align: center;"> Não Spam </th> <th style="text-align:right;background-color: white !important;text-align: center;"> Spam </th> </tr> </thead> <tbody> <tr> <td style="text-align:left;background-color: white !important;text-align: center;width: 3in; font-weight: bold;"> Não Spam </td> <td style="text-align:right;background-color: white !important;text-align: center;width: 3in; "> 267 </td> <td style="text-align:right;background-color: white !important;text-align: center;width: 2in; "> 8 </td> </tr> <tr> <td style="text-align:left;background-color: white !important;text-align: center;width: 3in; font-weight: bold;"> Spam </td> <td style="text-align:right;background-color: white !important;text-align: center;width: 3in; "> 195 </td> <td style="text-align:right;background-color: white !important;text-align: center;width: 2in; "> 530 </td> </tr> </tbody> </table> <table class="table table-bordered" style="font-size: 16px; width: auto !important; margin-left: auto; margin-right: auto;"> <thead> <tr> <th style="border-bottom:hidden;padding-bottom:0; padding-left:3px;padding-right:3px;text-align: center; color: red !important;padding-right: 4px; padding-left: 4px; background-color: white !important;" colspan="1"><div style="border-bottom: 1px solid #ddd; padding-bottom: 5px; ">p > 25%</div></th> <th style="border-bottom:hidden;padding-bottom:0; padding-left:3px;padding-right:3px;text-align: center; color: black !important;padding-right: 4px; padding-left: 4px; background-color: white !important;" colspan="2"><div style="border-bottom: 1px solid #ddd; padding-bottom: 5px; ">Observado</div></th> </tr> <tr> <th style="text-align:left;background-color: white !important;text-align: center;"> Predito </th> <th style="text-align:right;background-color: white !important;text-align: center;"> Não Spam </th> <th style="text-align:right;background-color: white !important;text-align: center;"> Spam </th> </tr> </thead> <tbody> <tr> <td style="text-align:left;background-color: white !important;text-align: center;width: 3in; font-weight: bold;"> Não Spam </td> <td style="text-align:right;background-color: white !important;text-align: center;width: 3in; "> 332 </td> <td style="text-align:right;background-color: white !important;text-align: center;width: 2in; "> 28 </td> </tr> <tr> <td style="text-align:left;background-color: white !important;text-align: center;width: 3in; font-weight: bold;"> Spam </td> <td style="text-align:right;background-color: white !important;text-align: center;width: 3in; "> 130 </td> <td style="text-align:right;background-color: white !important;text-align: center;width: 2in; "> 510 </td> </tr> </tbody> </table> <table class="table table-bordered" style="font-size: 16px; width: auto !important; margin-left: auto; margin-right: auto;"> <thead> <tr> <th style="border-bottom:hidden;padding-bottom:0; padding-left:3px;padding-right:3px;text-align: center; color: red !important;padding-right: 4px; padding-left: 4px; background-color: white !important;" colspan="1"><div style="border-bottom: 1px solid #ddd; padding-bottom: 5px; ">p > 50%</div></th> <th style="border-bottom:hidden;padding-bottom:0; padding-left:3px;padding-right:3px;text-align: center; color: black !important;padding-right: 4px; padding-left: 4px; background-color: white !important;" colspan="2"><div style="border-bottom: 1px solid #ddd; padding-bottom: 5px; ">Observado</div></th> </tr> <tr> <th style="text-align:left;background-color: white !important;text-align: center;"> Predito </th> <th style="text-align:right;background-color: white !important;text-align: center;"> Não Spam </th> <th style="text-align:right;background-color: white !important;text-align: center;"> Spam </th> </tr> </thead> <tbody> <tr> <td style="text-align:left;background-color: white !important;text-align: center;width: 3in; font-weight: bold;"> Não Spam </td> <td style="text-align:right;background-color: white !important;text-align: center;width: 3in; "> 410 </td> <td style="text-align:right;background-color: white !important;text-align: center;width: 2in; "> 73 </td> </tr> <tr> <td style="text-align:left;background-color: white !important;text-align: center;width: 3in; font-weight: bold;"> Spam </td> <td style="text-align:right;background-color: white !important;text-align: center;width: 3in; "> 52 </td> <td style="text-align:right;background-color: white !important;text-align: center;width: 2in; "> 465 </td> </tr> </tbody> </table> ] .pull-right[ <table class="table table-bordered" style="font-size: 16px; width: auto !important; margin-left: auto; margin-right: auto;"> <thead> <tr> <th style="border-bottom:hidden;padding-bottom:0; padding-left:3px;padding-right:3px;text-align: center; color: red !important;padding-right: 4px; padding-left: 4px; background-color: white !important;" colspan="1"><div style="border-bottom: 1px solid #ddd; padding-bottom: 5px; ">p > 75%</div></th> <th style="border-bottom:hidden;padding-bottom:0; padding-left:3px;padding-right:3px;text-align: center; color: black !important;padding-right: 4px; padding-left: 4px; background-color: white !important;" colspan="2"><div style="border-bottom: 1px solid #ddd; padding-bottom: 5px; ">Observado</div></th> </tr> <tr> <th style="text-align:left;background-color: white !important;text-align: center;"> Predito </th> <th style="text-align:right;background-color: white !important;text-align: center;"> Não Spam </th> <th style="text-align:right;background-color: white !important;text-align: center;"> Spam </th> </tr> </thead> <tbody> <tr> <td style="text-align:left;background-color: white !important;text-align: center;width: 3in; font-weight: bold;"> Não Spam </td> <td style="text-align:right;background-color: white !important;text-align: center;width: 3in; "> 443 </td> <td style="text-align:right;background-color: white !important;text-align: center;width: 2in; "> 112 </td> </tr> <tr> <td style="text-align:left;background-color: white !important;text-align: center;width: 3in; font-weight: bold;"> Spam </td> <td style="text-align:right;background-color: white !important;text-align: center;width: 3in; "> 19 </td> <td style="text-align:right;background-color: white !important;text-align: center;width: 2in; "> 426 </td> </tr> </tbody> </table> <table class="table table-bordered" style="font-size: 16px; width: auto !important; margin-left: auto; margin-right: auto;"> <thead> <tr> <th style="border-bottom:hidden;padding-bottom:0; padding-left:3px;padding-right:3px;text-align: center; color: red !important;padding-right: 4px; padding-left: 4px; background-color: white !important;" colspan="1"><div style="border-bottom: 1px solid #ddd; padding-bottom: 5px; ">p > 90%</div></th> <th style="border-bottom:hidden;padding-bottom:0; padding-left:3px;padding-right:3px;text-align: center; color: black !important;padding-right: 4px; padding-left: 4px; background-color: white !important;" colspan="2"><div style="border-bottom: 1px solid #ddd; padding-bottom: 5px; ">Observado</div></th> </tr> <tr> <th style="text-align:left;background-color: white !important;text-align: center;"> Predito </th> <th style="text-align:right;background-color: white !important;text-align: center;"> Não Spam </th> <th style="text-align:right;background-color: white !important;text-align: center;"> Spam </th> </tr> </thead> <tbody> <tr> <td style="text-align:left;background-color: white !important;text-align: center;width: 3in; font-weight: bold;"> Não Spam </td> <td style="text-align:right;background-color: white !important;text-align: center;width: 3in; "> 456 </td> <td style="text-align:right;background-color: white !important;text-align: center;width: 2in; "> 171 </td> </tr> <tr> <td style="text-align:left;background-color: white !important;text-align: center;width: 3in; font-weight: bold;"> Spam </td> <td style="text-align:right;background-color: white !important;text-align: center;width: 3in; "> 6 </td> <td style="text-align:right;background-color: white !important;text-align: center;width: 2in; "> 367 </td> </tr> </tbody> </table> ] --- # Curva ROC .pull-left[ <img src="01-intro-ml_files/figure-html/unnamed-chunk-58-1.png" style="display: block; margin: auto;" /> ] .pull-right[ <br/> <table class="table table-bordered" style="font-size: 20px; width: auto !important; margin-left: auto; margin-right: auto;"> <thead> <tr> <th style="empty-cells: hide;border-bottom:hidden;" colspan="1"></th> <th style="border-bottom:hidden;padding-bottom:0; padding-left:3px;padding-right:3px;text-align: center; padding-right: 4px; padding-left: 4px; background-color: white !important;" colspan="2"><div style="border-bottom: 1px solid #ddd; padding-bottom: 5px; ">Observado</div></th> </tr> <tr> <th style="text-align:left;background-color: white !important;text-align: center;"> Predito </th> <th style="text-align:left;background-color: white !important;text-align: center;"> Neg </th> <th style="text-align:left;background-color: white !important;text-align: center;"> Pos </th> </tr> </thead> <tbody> <tr> <td style="text-align:left;background-color: white !important;text-align: center;width: 3in; font-weight: bold;"> Neg </td> <td style="text-align:left;background-color: white !important;text-align: center;width: 3in; "> TN </td> <td style="text-align:left;background-color: white !important;text-align: center;width: 2in; "> FN </td> </tr> <tr> <td style="text-align:left;background-color: white !important;text-align: center;width: 3in; font-weight: bold;"> Pos </td> <td style="text-align:left;background-color: white !important;text-align: center;width: 3in; "> FP </td> <td style="text-align:left;background-color: white !important;text-align: center;width: 2in; "> TP </td> </tr> </tbody> </table> $$ \begin{array}{lcc} \mbox{TPR} & = & \frac{TP}{TP + FN} \\\\ & & \\\\ \mbox{FPR} & = & \frac{FP}{FP + TN} \end{array}` $$ ] .footnote[ [An introduction to ROC analysis](https://people.inf.elte.hu/kiss/11dwhdm/roc.pdf) ] --- # Curva ROC - Métrica AUC .pull-left[ <img src="01-intro-ml_files/figure-html/unnamed-chunk-60-1.png" style="display: block; margin: auto;" /> ] .pull-right[ <br/> <table class="table table-bordered" style="font-size: 20px; width: auto !important; margin-left: auto; margin-right: auto;"> <thead> <tr> <th style="empty-cells: hide;border-bottom:hidden;" colspan="1"></th> <th style="border-bottom:hidden;padding-bottom:0; padding-left:3px;padding-right:3px;text-align: center; padding-right: 4px; padding-left: 4px; background-color: white !important;" colspan="2"><div style="border-bottom: 1px solid #ddd; padding-bottom: 5px; ">Observado</div></th> </tr> <tr> <th style="text-align:left;background-color: white !important;text-align: center;"> Predito </th> <th style="text-align:left;background-color: white !important;text-align: center;"> Neg </th> <th style="text-align:left;background-color: white !important;text-align: center;"> Pos </th> </tr> </thead> <tbody> <tr> <td style="text-align:left;background-color: white !important;text-align: center;width: 3in; font-weight: bold;"> Neg </td> <td style="text-align:left;background-color: white !important;text-align: center;width: 3in; "> TN </td> <td style="text-align:left;background-color: white !important;text-align: center;width: 2in; "> FN </td> </tr> <tr> <td style="text-align:left;background-color: white !important;text-align: center;width: 3in; font-weight: bold;"> Pos </td> <td style="text-align:left;background-color: white !important;text-align: center;width: 3in; "> FP </td> <td style="text-align:left;background-color: white !important;text-align: center;width: 2in; "> TP </td> </tr> </tbody> </table> $$ \mbox{AUC} = \mbox{Area Under The ROC Curve} $$ ] **PS:** AUC varia de 0.5 a 1.0. O que significa se AUC for zero? .footnote[ [An introduction to ROC analysis](https://people.inf.elte.hu/kiss/11dwhdm/roc.pdf) ] --- # Curva ROC - Playground <a href = "http://arogozhnikov.github.io/2015/10/05/roc-curve.html"> <img src="static/img/roc_curve.gif" style=" display: block; margin-left: auto; margin-right: auto;"></img> </a> --- ## Ir para o R